Data Annotation Specialist: Unleashing the Power of Accurate Machine Learning Models
Unleash the power of your AI with Subul Impact Outsourcing’s data annotation specialists. Expertly annotated data for optimal machine learning results.
In the age of artificial intelligence (AI), there is one critical resource without which all the cutting-edge algorithms, quantum computers, and innovative AI models would be powerless: Data. And not just raw data, but accurately annotated data. This is where data annotation specialists, like the ones at Subul Impact Outsourcing & Hiring Social Enterprise, come into play.
The Vital Role of Data Annotation in Machine Learning
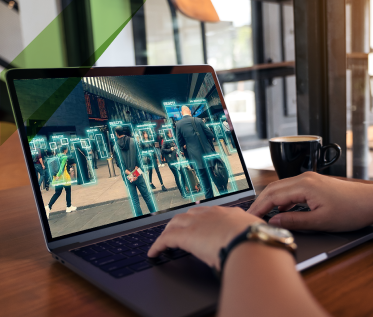
Machine learning is akin to teaching a child to understand the world around them. Just as a child learns to recognize a cat by being shown multiple examples of cats, an AI model learns to recognize patterns or make predictions by being fed large amounts of relevant, accurately labeled data.
Interested in learning more about our mission? Visit Our Story to discover how we are empowering disadvantaged communities and helping to build a more equitable world.
The Dependency of AI Models on Annotated Data
Without data annotation, there is no machine learning. If we want an AI to recognize a face, detect a disease from an MRI scan, or identify sentiment in a block of text, we need to feed it thousands, if not millions, of correctly labeled examples. Data annotation specialists perform this essential task, meticulously labeling or tagging data to train machine learning algorithms accurately.
Automated vs. Human Annotation: Balancing Cost and Accuracy
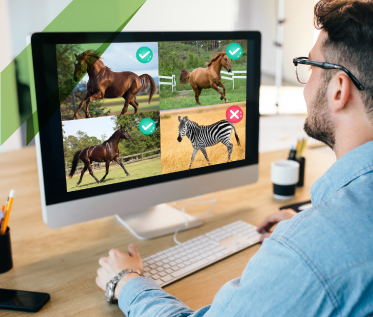
Just as there are many ways to teach a child, there are several ways to annotate data, each with its advantages and drawbacks.
Automated Annotation: Cost-Effective but Requires Investigation
Automated annotation involves the use of AI itself to label data sets. It can be a cost-effective method, especially for large data sets. For instance, we could download a thousand horse images from the internet and label them as ‘horse’ automatically.
However, automated annotation can risk accuracy. Suppose a few of those thousand images are not of horses but unicorns or zebras. In that case, the inaccuracies can hamper the effectiveness of the machine learning model trained on this data. This is where data annotation specialists prove invaluable.
Human Annotation: Higher Cost, but Unmatched Accuracy
While human annotation is generally more costly and time-consuming than automated annotation, it offers a level of accuracy that AI cannot yet match. Data annotation specialists can use their unique human capabilities of discernment and judgment to ensure the data’s correctness and reliability.
For instance, they can confirm whether a given image is a horse or not, and even classify it into specific breeds if they possess that level of expertise.
The Expertise of Data Annotators
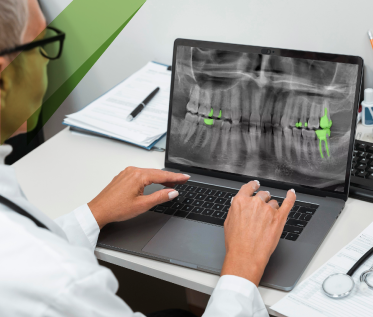
Just like different academic fields require different experts, different data types need different annotation specialists.
Annotating with Specificity: Leveraging Knowledge and Expertise
Data annotation specialists can use their expertise to annotate data with a high level of specificity. An expert in horse breeds, for instance, could label images with the specific breed of the horse depicted.
This level of detail can be invaluable when training more complex or specialized AI models.
Customization and Precision: Fine-Tuning Annotations for Optimal Results
The ability of human annotators to provide customized, fine-tuned annotations is another of their unique strengths.
They can draw polygons around the horse in an image to specify precisely which pixels are the horse, something automated tools often struggle with.
This level of precision is particularly crucial in fields like autonomous driving or medical imaging, where the smallest error can have grave consequences.
The Role of Expertise: Annotating Complex and Niche Data
Moreover, complex or niche data often require the expertise of trained data annotation specialists who understand the subject matter.
For example, annotating medical images requires knowledge of medical terminology and human anatomy.
As such, the role of data annotation specialists extends beyond mere labeling; they help transform raw data into a valuable resource that can drive AI advancements.
Striking the Balance: Specificity and Accuracy
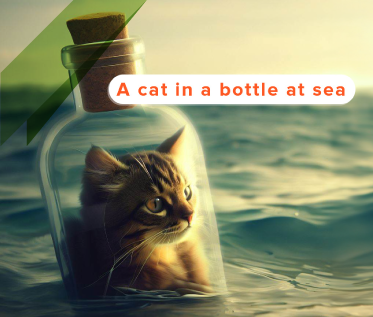
For effective machine learning, data must be annotated to both a degree of specificity and a degree of accuracy. The right balance depends on the machine learning problem at hand.
Annotating to a Degree of Specificity: Uncovering Detailed Insights
Specificity in annotation can yield detailed insights and allow AI models to make nuanced predictions. It’s the difference between knowing a picture depicts a horse and knowing it shows an Arabian horse galloping in a field at sunset. The more specific the annotation, the richer the data, and the more complex the tasks the AI can handle.
Ensuring a Degree of Accuracy: The Foundation of Reliable AI Models
However, specificity is meaningless without accuracy. A mislabeled data point can mislead the AI, leading to erroneous results. Therefore, ensuring the accuracy of annotations is a non-negotiable task for data annotation specialists. Their expert touch helps build the foundation of reliable, robust AI models.
The Significance of Problem Definition
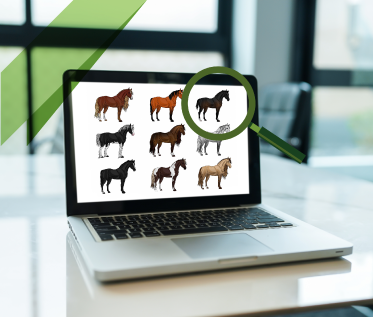
The problem definition, or the objective of the machine learning model, greatly influences the annotation process.
Problem Definition’s Impact on Annotation Needs
For instance, if you’re developing a general image recognition AI, labeling images as ‘horse’ or ‘not horse’ might suffice. But if you’re building an AI model for a specialist equestrian site, you’d likely need to label images with specific breeds, gaits, and other horse-related attributes. The problem definition sets the requirements for the depth and breadth of data annotation.
Aligning Annotation Approaches with Machine Learning Objectives
The methods used for data annotation should align with the objectives of the machine learning model. Simple models may be sufficiently trained with broad categories, while complex models may require detailed, specific annotations. It’s the job of a data annotation specialist to understand these needs and adapt their approach accordingly.
Adapting Annotation Strategies to Evolving ML Challenges
Moreover, as machine learning continues to evolve and tackle more complex tasks, data annotation needs to keep pace.
This is why at Subul, we prioritize continual learning and skill development for our data annotation specialists, preparing them to meet the ever-evolving challenges of AI training.
In conclusion, data annotation specialists like those at Subul Impact Outsourcing & Hiring Social Enterprise play a crucial role in the AI development pipeline.
By providing accurately annotated data, they empower AI models to learn, grow, and effectively solve complex problems.
Are you looking to enhance your AI models with accurately annotated data? Get a free trial with our expert data annotation specialists now!
Also, we invite you to learn more about our mission and the impact we are making at Subul. We are not just about delivering quality data annotation services; we are about empowering disadvantaged communities and helping to build a more equitable world.
Visit Our Story to find out more about our commitment to creating pathways to meaningful work and supporting sustainable practices.
Conclusion
In the ever-evolving landscape of AI, the role of data annotation specialists cannot be overstated. They help transform raw data into valuable insights that power sophisticated machine learning models, enabling them to understand our world and solve complex problems. At Subul Impact Outsourcing & Hiring Social Enterprise, we pride ourselves on our dedicated team of data annotation specialists, who combine technical expertise with a deep commitment to our social mission.
If you’re ready to unleash the full potential of your AI models with accurately annotated data, we’re here to help.